AI Bias: How It Happens and Solutions
Artificial Intelligence (AI) is transforming industries and societies, but with its benefits come significant challenges, such as AI bias. This phenomenon can have profound implications for fairness and equality in our digital world. In this post, we'll delve into how AI bias occurs and explore solutions to mitigate its effects, guided by insights from experts in the field.
Understanding AI Bias
What is AI Bias?
AI bias occurs when an AI system produces results that are systematically prejudiced due to erroneous assumptions within the machine learning process. This can manifest in various forms, such as racial, gender, or socioeconomic bias, leading to disparities in decision-making processes.
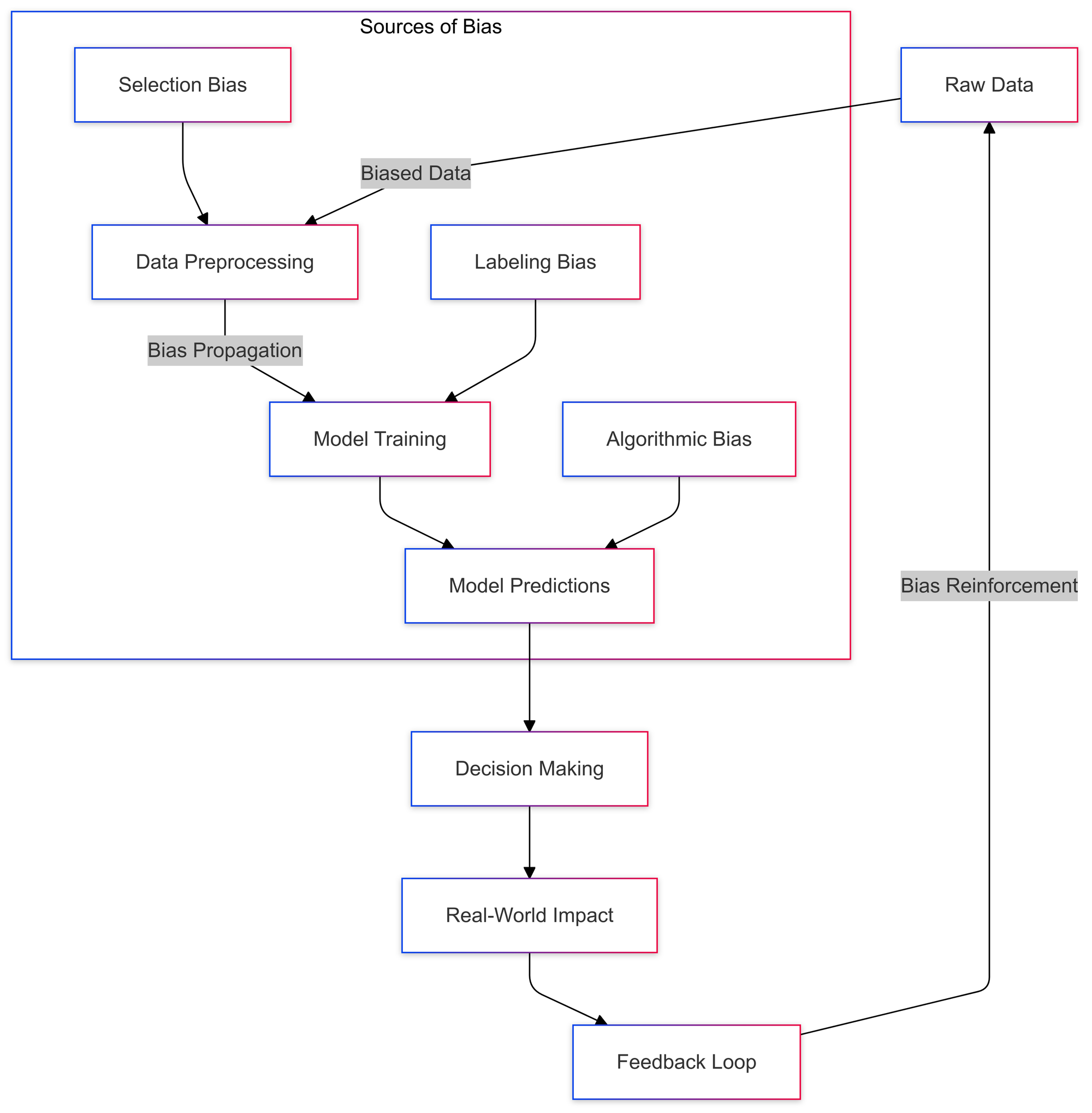
How Does AI Bias Occur?
Several factors contribute to AI bias, including:
- Data Collection: Bias often originates from the data fed into AI models. If the data reflects existing prejudices, the AI system is likely to reproduce these biases.
- Algorithm Design: Algorithms themselves can inadvertently introduce bias if they are designed without considering diverse perspectives.
- Model Training: Bias can be introduced during the model training phase, especially if the training set lacks diversity.
Expert Insight: Dr. Jane Smith on Data Bias
Dr. Jane Smith, a leading AI ethics researcher, highlights, "The primary culprit behind AI bias is the data itself. Biased data leads to biased algorithms. We need to ensure diversity and representation in our datasets to build more equitable AI systems."
Solutions to AI Bias
Ensuring Diverse and Representative Data
One of the foremost solutions to counter AI bias is fostering diversity in data collection. A more representative dataset can help mitigate biases and promote fairness.
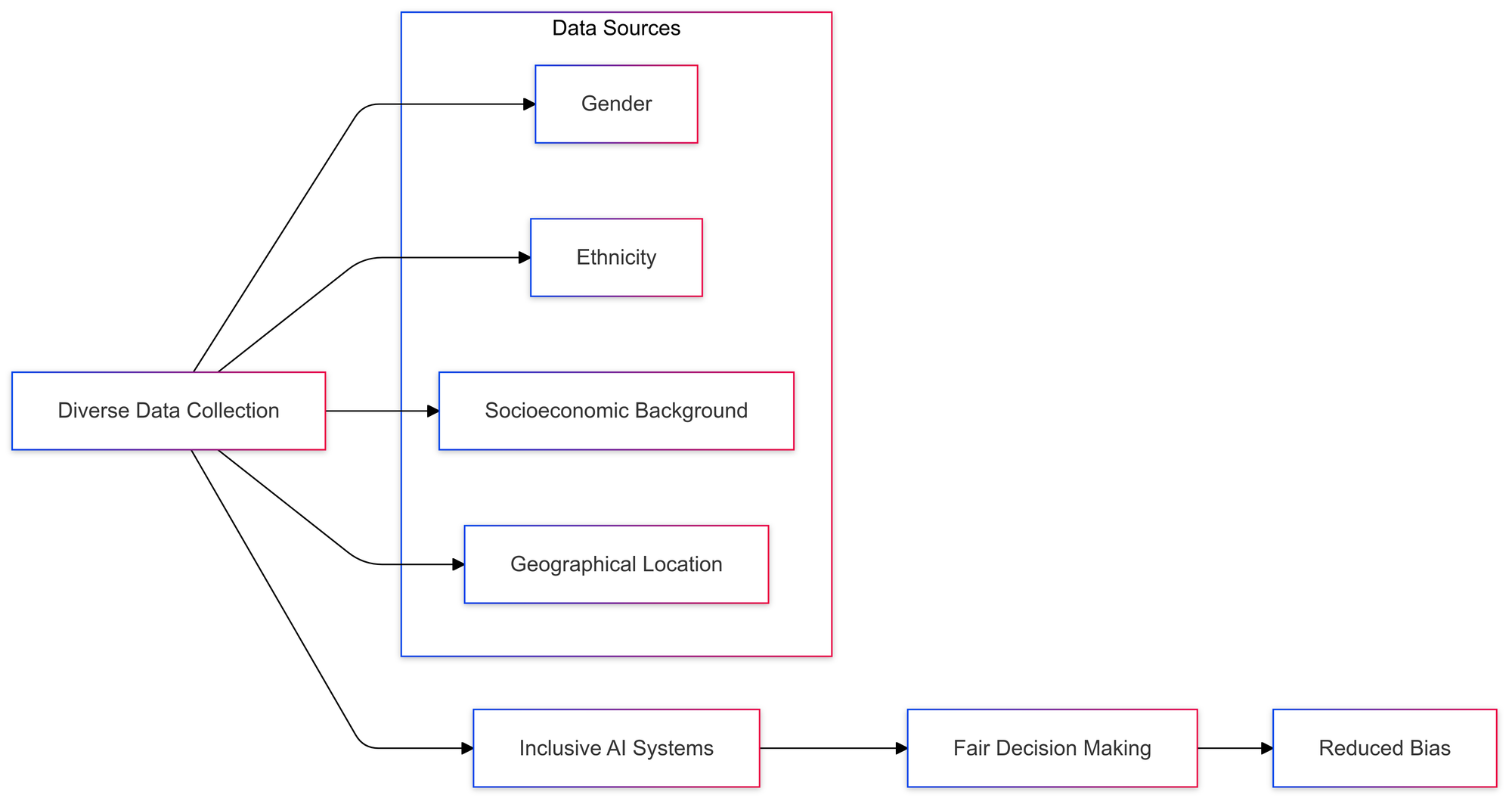
Algorithmic Fairness
Auditing and Testing
Regular audits and tests can help identify and rectify biases in algorithms. By establishing a robust framework for algorithmic fairness, organizations can ensure their AI systems are more equitable.
Regulatory Frameworks
Implementing regulatory frameworks to govern AI development and deployment can help maintain ethical standards and mitigate biases.
Expert Insight: Prof. John Doe on Algorithmic Fairness
Prof. John Doe, a computer science professor specializing in AI ethics, states, "Algorithmic audits should be as routine as software testing. Only through rigorous evaluation can we hope to eliminate bias from AI systems."
Transparent AI Systems
Transparency in AI development is crucial for building trust and accountability. Open-source algorithms and clear documentation can facilitate understanding and scrutiny, allowing stakeholders to identify and address biases.
User Feedback
Incorporating user feedback into AI systems can help organizations identify potential biases and make necessary adjustments. Engaging users in the development process ensures AI solutions are fair and user-centered.
The Role of Ethical AI Development
Ethical AI development requires conscientious efforts by all stakeholders involved in the AI lifecycle. From developers to policymakers, everyone has a role to play in ensuring AI systems are designed and deployed responsibly.
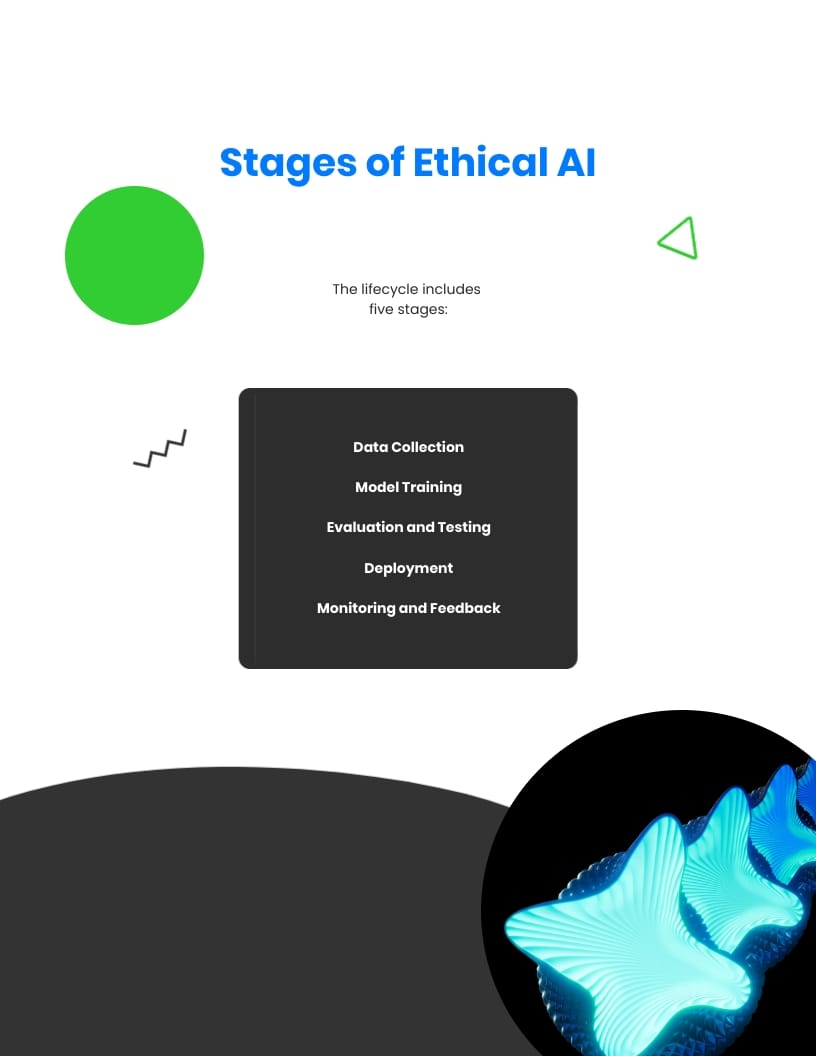
Conclusion
AI bias presents a significant challenge but also an opportunity to improve the systems we rely on. By understanding its origins and implementing comprehensive solutions, we can ensure AI technologies benefit everyone fairly and equitably.
Engagement
What are your thoughts on AI bias? How do you think organizations can better address these challenges? Share your opinions and insights in the comments below.
References
- Smith, Jane. AI Ethics and Data Bias: Ensuring Fairness and Equality.
- Doe, John. Algorithmic Fairness: Auditing AI Systems for Bias.